We are pleased to announce that our researcher, Giulio Meucci, received the NATO SET Panel Best Paper Award for the publication “Point Cloud Transformer (PCT) for 3D-InISAR Automatic Target Recognition applied to real data“.
This research demonstrates the remarkable potential of AI in the radar field, showing that classifiers trained on purely synthetic data can achieve impressive accuracies on real, never-before-seen 3D-InISAR data.
Abstract:
Three-Dimensional Interferometric ISAR imaging is an effective technique to overcome the interpretation issues related to the classical bidimensional ISAR imaging. As a matter of fact, the bidimensional technique produces images that strictly depend on the geometry of the whole radar-target system and on the relative motion between radar and target. This poses some limits on the use of Automatic Target Recognition (ATR) systems. As it has been shown in literature, the three-dimensional approaches lead to a more complete and reliable representation of the target, that can be more easily fed to a classification system.
Among all the possible Machine Learning methods, Transformer models have attracted widespread attention in multiple areas like Natural Language Processing, Object Detection and 3D point cloud processing, achieving impressive results in various 3D tasks. Since the acquisition system will output an unknown number of points in a random order, we identified a classifier, namely Point Cloud Transformer (PCT), able to process a variable number of input elements.
PCT is trained on an ad-hoc generated 3D dataset, which in this preliminary experiment contains three different target types: cars, tanks and military trucks. The goal of this work is to show the performance of the transformer-based classifier when applied to real data obtained applying the 3D-InISAR procedure to the multi-aspect and fully polarimetric turntable dataset of the T72 tank, publicly available at SDMS-AFRL website. In particular, the real point clouds are extracted using different 3D-InISAR techniques and polarization, explicitly: Coherence-based, SPAN, VV-HH, VV and HH.
The first results showed over 90% accuracy on the point clouds extracted from the real data, validating therefore the possibility of training an accurate classifier without the need of a large real dataset. After an initial assessment of the performance of the network trained on the synthetic dataset we apply the transfer-learning technique to increase the classification accuracy.
Authors:
G. Meucci1,2, F. Mancuso1,2, S. Ghio1, E. Giusti1, M. Martorella1,3
1National Laboratory of Radar and Surveillance Systems (RaSS) – CNIT, Galleria Gerace 14 56124 Pisa ITALY
2University of Pisa, Department of Information Engineering – Pisa, ITALY
3 University of Birmingham, Department of Electronic, Electrical and Systems Engineering – Birmingham
Some details about the SET:
SET-318 Specialists’ Meeting on “Artificial Intelligence (AI) / Machine Learning (ML) for Cognitive Radar (CR)”
organized by the Sensors and Electronics Technology Panel
date: 15-16 April 2024
Amsterdam.
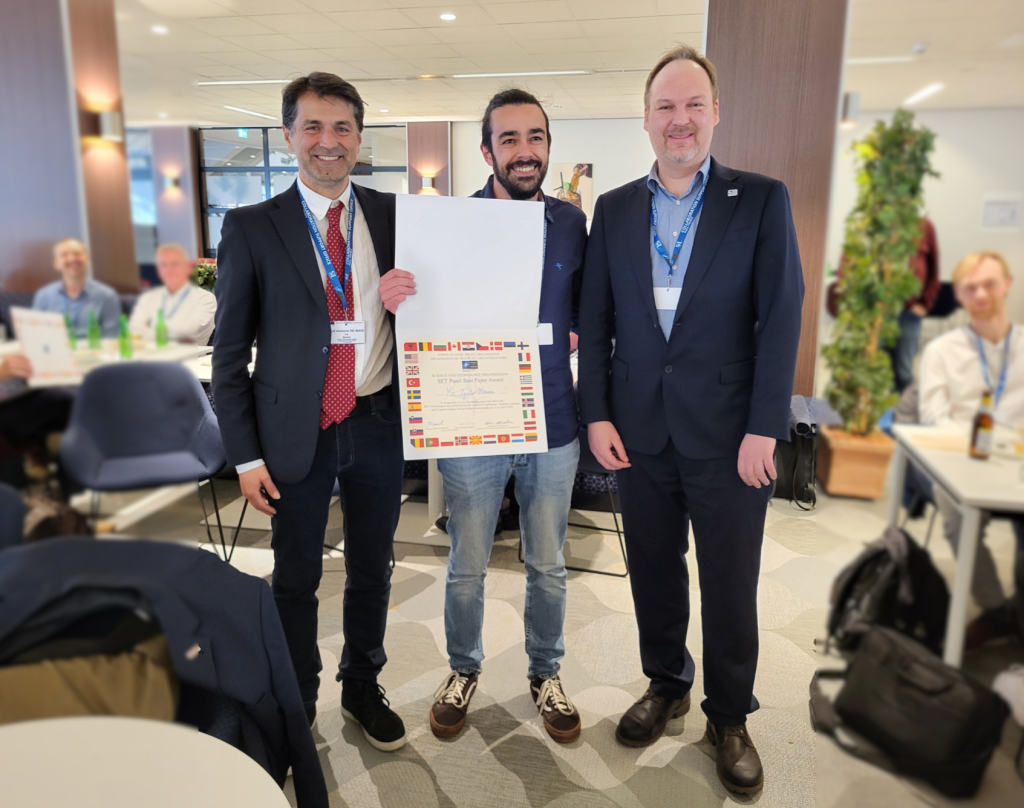